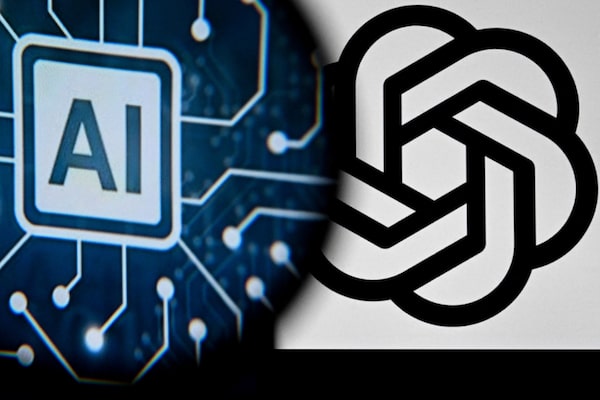
San Francisco-based OpenAI kicked off a wave of generative AI hype when it released ChatGPT in November, 2022.KIRILL KUDRYAVTSEV/Getty Images
The corporate world has been enthralled with generative artificial intelligence for more than a year, as the technology has advanced in leaps and bounds. But the performance gains in large language models, which can produce and summarize text, could be starting to slow down. The technology developed by leaders in the space, such as OpenAI, Google, Cohere and Anthropic, may not ultimately be that unique either, suggesting competition is going to become a lot more intense.
“The market may not be as big as expected and margins may be thin because there is no real moat,” said Gary Marcus, an emeritus professor of psychology and neural science at New York University who has started two AI companies. “Everybody is building more or less the same technology, scraping the same data.”
San Francisco-based OpenAI kicked off a wave of generative AI hype when it released ChatGPT in November, 2022. Powering the chatbot is a large language model, or LLM. Earlier versions of LLMs produced text passages that were rambling and borderline incoherent, but today’s models are impressively fluent.
The release of Google’s latest suite of LLMs in December, which it calls Gemini, shows some of the challenges of making further progress. Researchers use a series of benchmarks to gauge LLMs on their ability to reason, translate text and answer questions, among other tasks. Google’s report said that its most capable Gemini model was “state-of-the-art” on 30 out of 32 measures, beating out OpenAI, whose GPT-4 model is generally considered the most capable.
But Google did not beat OpenAI by much. The most capable Gemini model outperformed GPT-4 by just a fraction of a percentage point in some cases. For some AI observers, this was a surprise. Google, with its history of AI breakthroughs, legions of staff and immense computing power, didn’t exactly blow a chief rival away. The results also raise the question of whether LLMs will become commoditized, which refers to the process by which a good becomes indistinguishable from its competitors.
Other challenges remain, too, such as the propensity of LLMs to hallucinate and make things up. Generative AI companies are also facing legal problems over training on copyrighted material. Striking licensing deals with content providers is one solution, but could weigh on profit margins.
“Companies in this space are probably overvalued,” Mr. Marcus said. “We may see a recalibration in 2024 or 2025.”
Much of the advancement in LLMs has been due to scale: huge amounts of training data paired with loads of computing power to build very big models with billions of parameters or nodes, which is a measure of the complexity of the model.
“If you spoke to anyone in April of 2023, people were talking about OpenAI working on GPT-7 and how it’s going to be a trillion nodes, and it’s going to be sentient intelligence,” said Alok Ajmera, chief executive at financial technology company Prophix Software Inc. in Mississauga. “What’s happened is there’s marginal return by increasing the number of nodes. More computer power and more data to train isn’t helping the large language model come up with more interesting things.”
That’s not to say progress is ending, of course. The general principle behind scaling with data and computing power is still true, said Jas Jaaj, managing partner of AI at Deloitte Canada, but the gains are not happening at the same pace. “The rate in which the efficiency and the performance of the models is going up is now relatively slowing down,” he said.
Meanwhile, the number of LLMs available for corporate customers to use is only increasing. Not only are there proprietary developers such as OpenAI, there is an entire ecosystem of open-source LLMs that can be free to use for commercial purposes. There are new entrants, too, such as France-based Mistral AI, which was founded only last year. Meta Platforms Inc. has released its LLMs into the open-source community and in December partnered with International Business Machines Corp. and other companies to promote open-source development.
Companies using generative AI are hardly beholden to a single provider today, since swapping one LLM for another can be fairly straightforward. “We don’t want to get vendor lock-in when it comes to developing these things,” said Ned Dimitrov, vice-president of data science at StackAdapt, a Toronto-based programmatic advertising company that is testing generative AI. “It’s an evolving field, so if something open-source becomes available tomorrow that performs better, it should be very easy to switch.”
Meta’s open-source push, he said, is an attempt to ensure there are lots of models available so that rival tech giants don’t control the market with proprietary technology. “That’s a very strategic play, where they want to make it commoditized,” he said.
If that happens and performance levels out, developers of LLMs will have to compete on different attributes for customers. Toronto-based Cohere, for example, emphasizes the privacy and security benefits of its technology, which is important for business users. Indeed, Canadian business leaders surveyed by Russell Reynolds Associates recently said data security and privacy concerns are the top barrier to deploying generative AI.
Cost is emerging as another important factor. Here, open-source models have the advantage. “That’s one of the reasons we’re looking to leverage, in some cases, open-source platforms. This way we can pass some of these savings on to our customers,” said Muhi Majzoub, chief product officer at Open Text Corp. The Waterloo-based tech company rolled out a suite of AI products this month, including a productivity tool for document summarization, conversational search and translation.
Many other Canadian companies are opting for open-source models. According to a recent IBM survey, 46 per cent of companies that responded are experimenting with open-source technology, compared with 23 per cent using tech from an outsider provider and 31 per cent developing tools in-house. “What open-source is doing is really giving you scale and speed to market,” said Deb Pimentel, general manager of technology at IBM Canada. Still, Ms. Pimentel expects that companies will take a hybrid approach, and use a mix of different technologies.
While the range of LLMs available today may pose competitive challenges to the companies that build them, the situation is ideal for companies looking to take advantage of generative AI. “I don’t think we’re at a point where an organization should put all their eggs in one basket, because it’s too early to say that there’s a clear winner,” said Mr. Jaaj at Deloitte. “Our recommendation to organizations is: Work with multiple players.”